About
Leveraging deep learning (LSTM) to analyse sequence of events that have occurred in a customer's life time in order to anticipate their financial needs and offer personalized recommendations
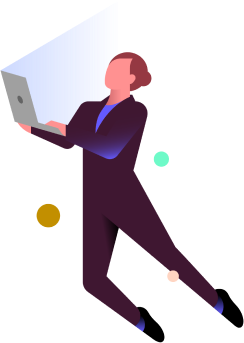
Leveraging deep learning (LSTM) to analyse sequence of events that have occurred in a customer's life time in order to anticipate their financial needs and offer personalized recommendations
Enhancing Small and Medium Enterprises with streamlined financial solutions.
Imagining banking in the future on Apple Vision Pro!
Protecting vulnerable customers from fraudsters through smart fraud controls
HSBC Kinetic is a mobile banking app designed for the everyday financial needs of small business owners. The app uses...
Marc Du Plessis, the Executive Head of the Looksee Platform at Standard Bank, presents a product that has made a...
Following the announcement of its company purpose in June 2021, the French bank La Banque Postale (LBP) became a mission-led...
Discover the evolving landscape of cloud technology in banking, as discussed in a recent session hosted by Qorus and Infosys...
Insurers are increasingly using AI and data to transform the way they operate. Laura Barkatz, Global Lead, Data Strategy, and...